Stress is one of the more prevalent mental disorders found in today’s society. A recent British survey found that as many as 74% of the population felt that they had felt “so stressed at some point over the last year they felt overwhelmed or unable to cope”. To make matters worse, as many as a third of respondents said that they had experienced suicidal thoughts as a result of stress, and 16% reported that they had actually performed self-harm as a consequence of stress. In a forthcoming publication, Neurons worked with the University of Southern Denmark to create mobile biometric and neurometric markers of stress. Here, we present some of the main results from what is to be presented at the HCI International 2020 conference.
The problem with stress
There is little doubt that stress has a major influence on our society. Stress goes much beyond being a psychological phenomenon. Instead, sustained stress is well-known to be associated with a host of other physiological, neural and mental changes such as ulcers, brain changes, and cognitive changes. Thus, stress is also associated with a host of different diseases and disorders, such as mental health problems, cardiovascular disease, obesity, menstrual problems, sexual dysfunction, skin and hair problems, and gastrointestinal problems. Stress is a disorder in sore need of a cure.
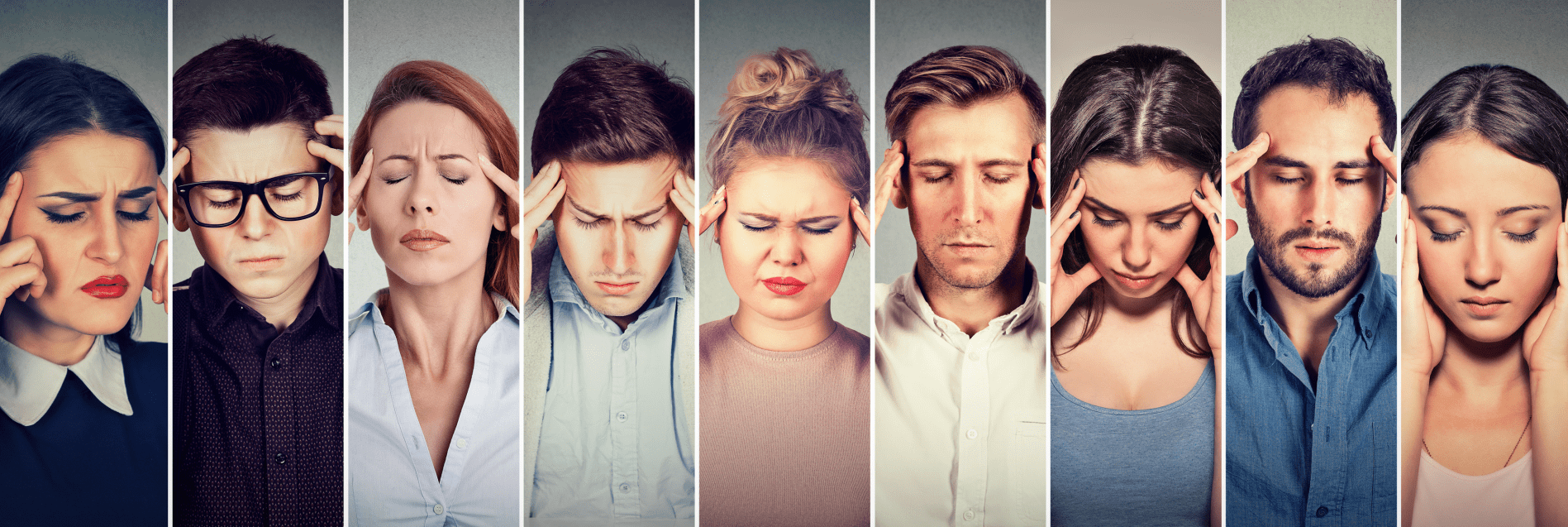
With these issues at stake, intervention towards stress is a crucial goal for any society. Here, we can distinguish between three main steps in an intervention:
- Detection -- our ability to reliably detect and even predict stress either before or while it is happening, is a crucial step.
- Assessment -- having reliable methods and metrics allow us to qualify and quantify stress. This is crucial as we measure both the severity of stress and any change in stress during interventional programs.
- Interventions -- based on previous experiences and theoretical developments, we can conduct different types of interventions that attempt to both prevent, reduce and treat stress and the related adverse effects of stress.
Together, these steps are thought to provide the main steps in stress prevention and treatment. However, how each step is implemented can vary from approach to approach.
Stress at the workplace
As we spent major time in our everyday lives in the workplace, work-related stress (WRS) has been studied for decades. The World Health Organization (WHO) characterizes WRS as:
“(...) the response people may have when presented with work demands and pressures that are not matched to their knowledge and abilities and which challenge their ability to cope.”
A recent study also demonstrated that the costs of WRS are raging sky high. In the study, performed by Hassard and colleagues, data was collected from Australia, Canada, Denmark, France, Sweden, Switzerland, the United Kingdom, the United States, and the EU-15. Crucially, the study found that work-related stress in 2014 across these countries was sizeable and ranged from US$221.13 million to $187 billion annually. The WDS related costs were found to be mainly driven by productivity losses (between 70 to 90%), while healthcare and medical costs accounted for the remaining 10% to 30%.As such, workplace stress can be considered an important target for stress intervention efforts. Here, recent scientific and technological advances can be included to alleviate stress: improvement in our ability to measure stress using neuroscience methods, and the rise of consumer electronics that allow portable solutions.
Scientific advances in measuring stress
Stress has been studied for decades, but recent accounts have started to use neuroscience tools to better understand the brain bases of stress. A number of studies have been performed, suggesting that we are getting closer to a deeper understanding of the mechanisms underlying stress. While the bodily responses have been understood in detail for many years but our understanding of how exactly the brain responds during stress has still been more difficult to untangle.
Still, recent accounts have come up with tangible findings that can be sought to be reproduced. Here, we focus on four main hypotheses, and briefly explain the main findings behind them:
- Lewis et al. (2007) found that increased stress was related to increased right-sided frontal brain dominance, as measured by frontal alpha EEG data.
- Seo & Lee (2012) reported that stress was related to an increase in the summation of frontal activity in the beta range.
- Minguillon et al (2016) found that stress was related to an increase in the relative frontal engagement in the gamma frequency range.
- Stress is related to increased heart rate variability, as shown by Taelman et al (2009) and Kim et al. (2018), although the relationship between stress and HRV is thought to be more complex.
The rise of consumer electronics and “quantified self”
In recent years, there has been an explosive growth in the number of wearable digital devices that can track different biometric signals. At the same time, IT costs are getting substantially lower, from the electronic devices to the storage of large amounts of data. Together, this paves the way for a true degree of “quantified self” in which wearable digital devices can be used to track our every move, both physically and physiologically.As a part of this revolution, new devices are made available, ranging from the dedicated GPS tracking, and heart rate monitoring of wearable bracelets to the recent skin conductance found in Apple Watches, and all the way to headbands that measure the electrical discharges from the brain, such as the Muse headband, primarily used for meditation and mindfulness purposes.
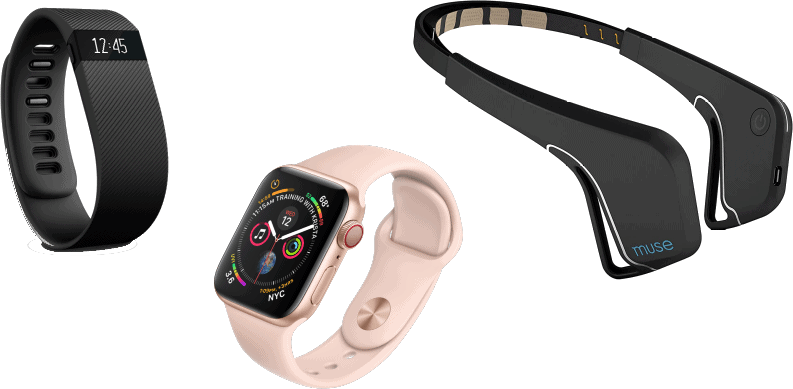
Study aims
The aim of the present study is twofold:
- To test whether it is possible to reliably measure stress as it unfolds in work-related situations
- To test whether it is possible to measure stress using lightweight “consumer electronic” wearable devices
Should this study be successful, it will mean that we can possibly have a new toll in stress intervention. Provided it is successful, a wearable measure of stress can allow early detection and thereby interventions that have hitherto been impossible, and on a scale that until now has been impossible. Based on this, we have formed two classes of methods -- first, hypothesis testing that aims to test previous findings, and second, exploratory analyses that test whether it is possible to reliably measure and predict stress from the data. Each approach is applied to both our standard neuroimaging measure (ABM EEG) and consumer-grade equipment (Muse headband). Based on the previous studies reviewed, our first step is to test whether these findings can be confirmed using our methods (ABM and Muse). The hypotheses are:
- H1: Stress is related to a relative increase in right-dominant frontal asymmetry
- H2: Stress is related to an increase in frontal beta
- H3: Stress is related to increased frontal relative gamma
- H4: Stress is related to decreased heart rate variability (HRV)
Each hypothesis is then tested by the method as described by each article, following their procedures to the point.
As part of the study, we also gather an immense amount of data, even on a participant level. This allows us to explore the data for generalized trends that predict the level and strength of stress from the data. Here, we employ different types of previously proven exploratory methods to test whether they can predict stress levels from the data. These methods include:
- Least absolute shrinkage and selection operator (LASSO)
- Least-angle regression (LARS)
- Partial Least Squares (PLS) regression
- Machine Learning (Tensorflow keras)
These methods are powerful analysis tools allowing for the detection of signals in the data that reliably predict stress levels.,
Brief methods
In total, we recruited and tested 63 participants (age mean 30.9, st.dev = 5.5, 32 women). Each participant was equipped with both an Advanced Brain Monitoring (ABM) X10 EEG brain monitor and a Muse headband EEG headset. Via the ABM headset, we also recorded heart rate, which was then used to calculate heart rate variability (HRV). Participants were positioned in front of a 27 inch computer monitor, and with a 14 inch second screen to their left side. The computer was running OpenSesame v3.2.7 in a Windows 10 environment. Most stimuli occurred on the screen in front of the participant, but during the stress test, a documentary was continuously shown on the secondary screen.
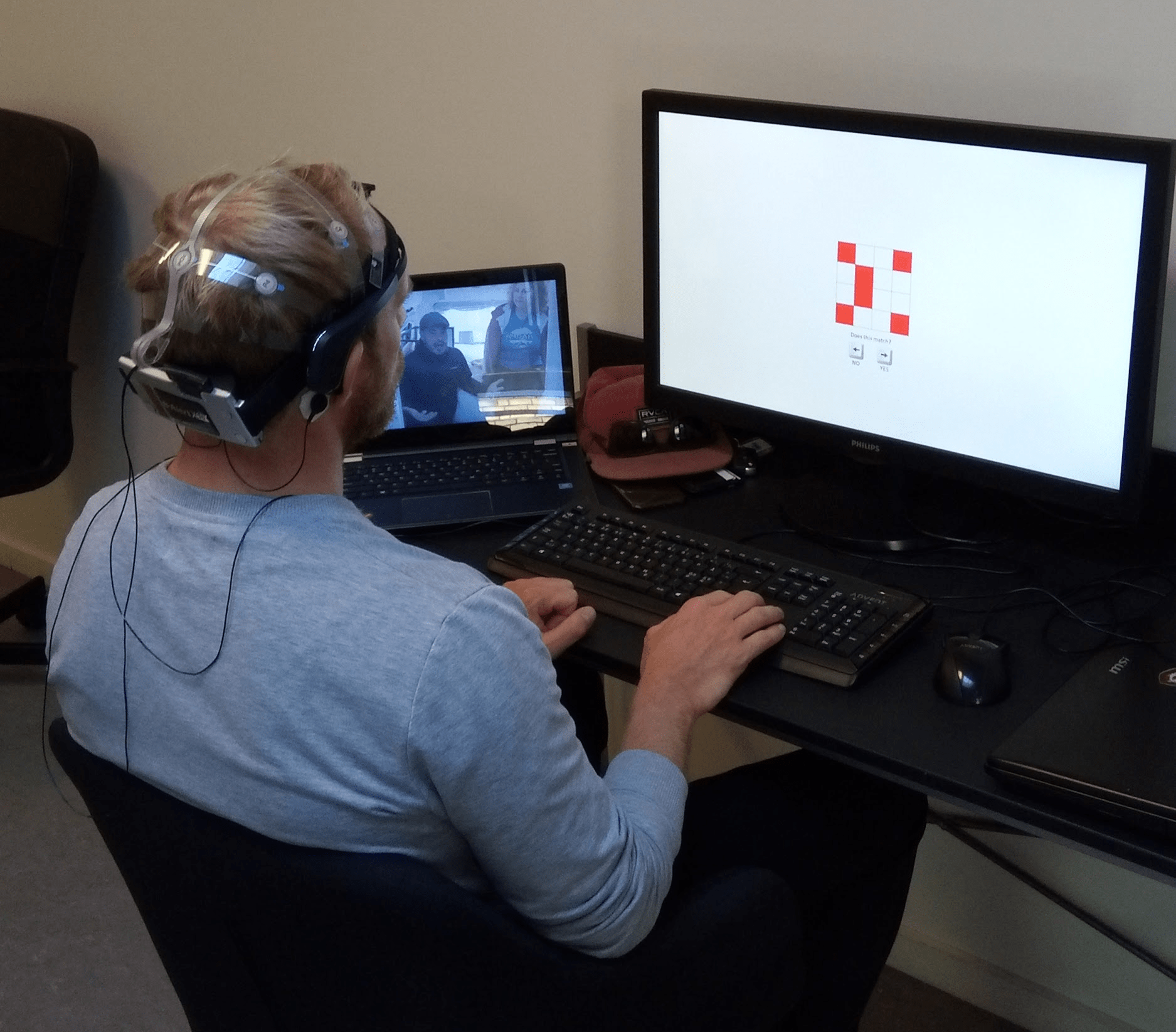
Stress manipulation
The trial was conducted in the same sequence for all participants:
They were first given a set of different tasks such as watching a documentary, doing tasks related to arousal and working memory. This was not directly related to the study, but helped to make the participants acquainted with the study setup and at the same time spend a few mental resources before the stress task started.
During the stress phase of the trial, we used three load levels: We had “Rest” phases between each task level.
- In “Stress1” participants were asked to watch a documentary, and to pay attention to the content.
- In “Stress2” they were asked to pay attention to the documentary while doing a memory task (They were presented to 3 digits and after 4 seconds they were asked to recite the digits in the same order).
- In “Stress3” participants were asked to pay attention to the documentary while two memory tasks (They were presented to a pattern then 3 digits to remember, and after 4 seconds, they were asked to answer a question about the pattern and recite the digits in the same order).
At the end of the trial, we included a final “Rest” phase.
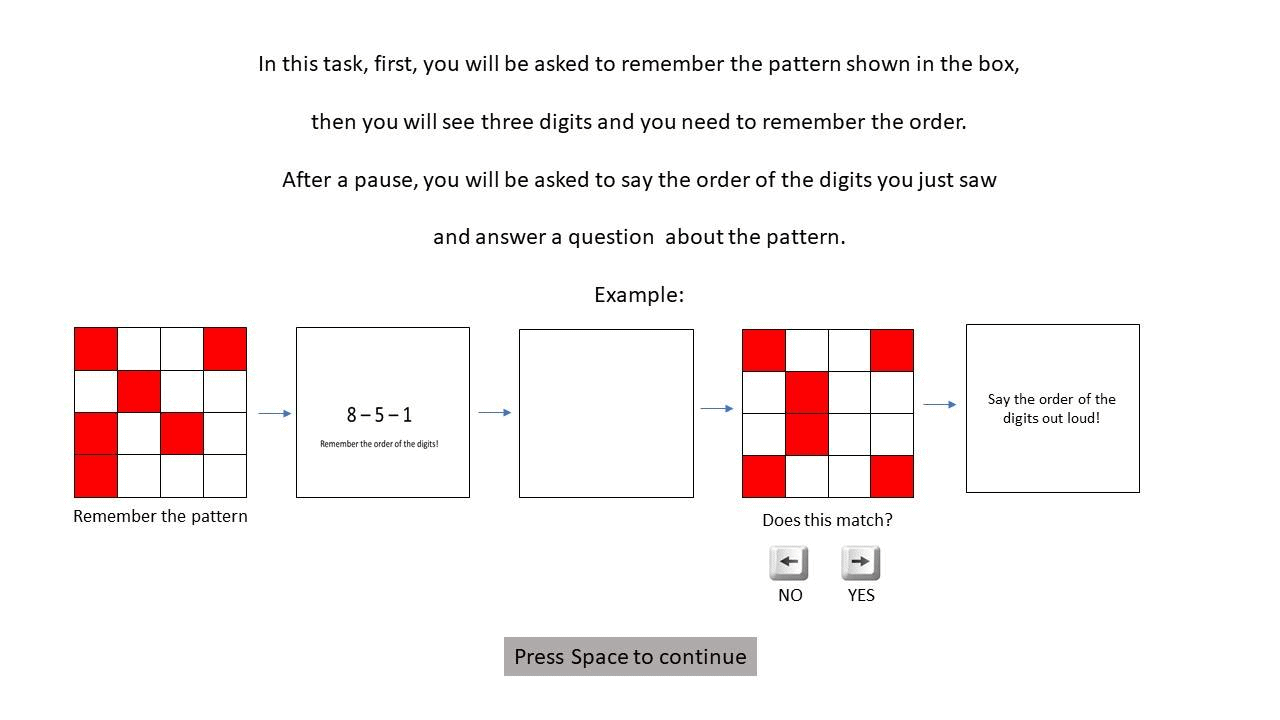
After the trial, participants were unmounted with the equipment and given an online survey to complete. In this survey, they were asked about their experience of stress during each of the stress tasks and asked to rate their level of experienced stress using a 7-point Likert scale ranging from 1 (“not stressed at all”) to 7 (“extremely stressed”).
Results: prior research works but AI dominates!
In running the study, we made two main types of analyses. First, we tested whether we could confirm findings from previous studies. Second, we ran exploratory analyses including machine learning approaches to test whether we could produce scores that were more reliable predictors of stress than previously published approaches.
Testing prior studies
Our study confirms all prior studies of the brain bases of stress. Indeed, we can confirm that stress is related to a change in the frontal brain asymmetry in the alpha band (H1), that frontal beta increases with stress (H2), and that frontal relative gamma increases with stress (H3). However, although we could confirm that heart rate variability was related to stress (H4) we found that stress was associated with increased variability, not decreased as previous studies have suggested.However, we also find that despite these promising findings, all hypothesis-driven metric except one performed sub-par on being reliable metrics for stress. No brain-derived metric proved to be much better than chance at estimating whether a state was stress or rest. Instead, HRV proved to have a very high prediction rate, with an accuracy of no less than 78%!
We then performed exploratory analyses to test whether the data power found in EEG data could be used to create a strong stress predictor. Here, we found that the best performing EEG metric was when using a deep machine learning model trained on the ABM EEG data -- this produced a prediction accuracy of no less than 82%, which is close to what is considered to be sufficiently accurate for clinical diagnoses (90%). More recent analyses have improved these numbers further and will be included in the full paper.Interestingly, despite being a consumer-grade tool, the Muse headband produced a relatively reliable score for predicting stress, with a classification accuracy of 72%. While this is a promising result, it is still poorer than HRV and ABM based metrics. Moreover, as the Muse headband lost many data points, and only a few respondents could be used for the calculations, this puts a serious restriction on whether the method can be used to reliably assess stress levels.
Conclusion
In sum, we believe that the results of this study have demonstrated the following:
- That stress is a brain-related response or state that can be reliably tracked using brain monitoring systems.
- That prior studies have detected parts of the brain mechanisms responsible for stress, but that these are far from the full picture.
- Exploratory methods, even when used conservatively, can identify stress with a high degree of accuracy.
- Even consumer-grade tools such as EEG headbands and especially HRV devices can prove to be highly scalable measures of stress.
Together, this suggests that we are homing in on a better understanding, prediction, and assessment of stress levels -- something that is to be considered some of the most important steps in stress intervention.We expect that a few natural next steps should be taken:
- Validation of the stress metrics (ABM, Muse, HRV) in work-like situations
- The creation of a stress-based biomonitor for companies and society at large
- Further work on improving the stress metric to provide even better prediction accuracy, possibly reaching even clinical degree status
To get more information, stay tuned to our insights section. And if you're interested in running a test that involves stress, just reach out!