The ability to measure emotions as they unfold over time has always been one of the hallmarks of neuromarketing and consumer neuroscience. After all, there are tons of reasons as to why we don’t ask participants “how do you feel now?” every second. But as traditional emotion metrics can always be improved, new classification methods be tried out. In a recent IEEE paper, we demonstrate how machine learning can be used to identify the two main dimensions of emotions in EEG signals: arousal and valence.
Dimensions of emotions
In neuromarketing, emotions are all the rage. Measuring feelings just won't cut it, as the direct and subconscious responses in the brain and body tell much more nuanced stories of how we respond. In consumer research, it's been hard enough to demonstrate that emotions are different than feelings. The two words are often used interchangeably and leading to much confusion in discussions. So let's set the record straight: "emotions" are direct and subconscious brain/body responses to events, while "feelings" are the conscious experience we have of some of these responses. Put this way, many emotional responses can lead to feelings, but you can't have feelings without emotions.
Many emotional responses can lead to feelings, but you can't have feelings without emotions
Second, while we still struggle to determine exactly what feelings are and how many dimensions there really are, emotions are typically more simple. We tend to distinguish between two dimensions of emotions: arousal and direction (or valence, or motivation). Arousal responses signify the intensity of emotions. You can see this in the change in respiration and pulse, in the dilation of the eyes' pupils, and sometimes in a momentary "freezing" of the body. Arousal responses like these happen for both negative and positive events, and therefore we need to have another way to discern the value, or valence, of emotional responses.
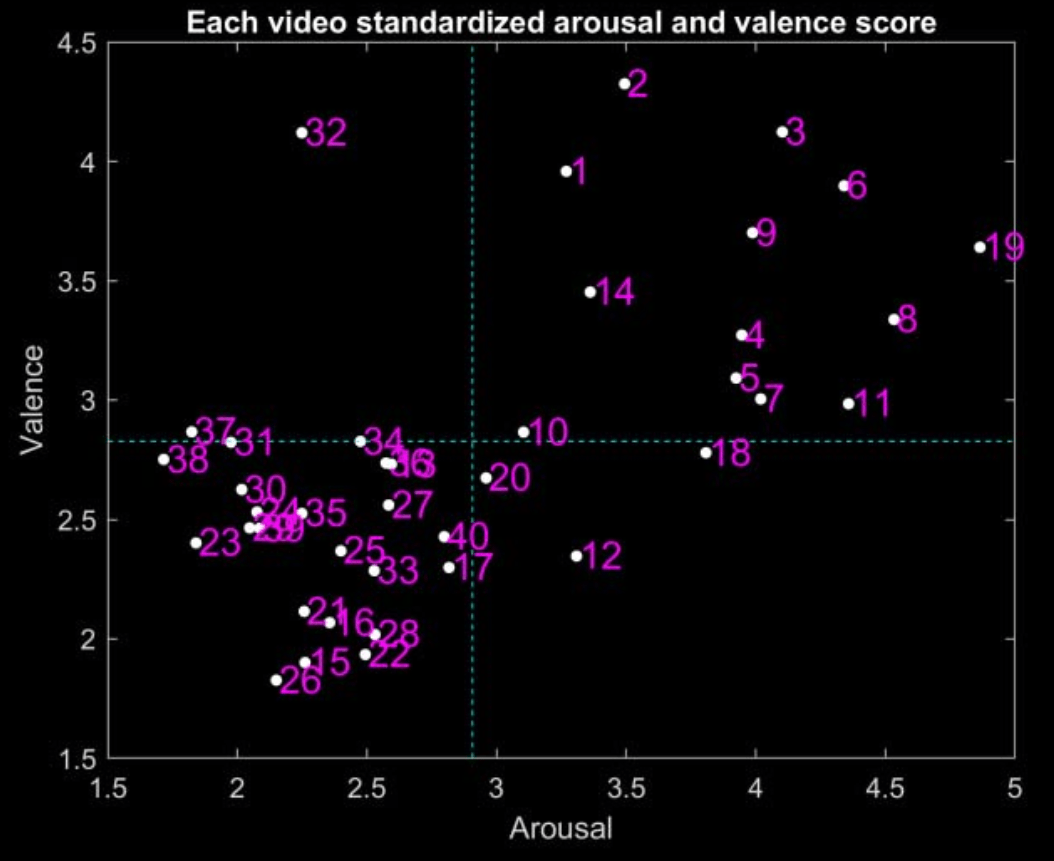
There are two ways to measure the value of emotional responses. One way is to use a straightforward notation of whether the experience is positive or negative. This is often called valence. A second term is closer to the original meaning of the term emotions (emovere is Latin for the term "to move"). As we know, emotions are not just passive reflections, but they lead to active changes in the organism; from small changes in attention to behavioral movements.
Therefore, another way to portray emotional value is the change in action they lead to. Here, we discern between approach and avoidance behaviors. That is, some emotions lead you to pursue, choose and approach something, while other emotions make you look away or directly avoid something. Think about how you are more likely to pick up a high-calorie item when hungry, and how you avoid a dangerous-looking dog.
Predicting emotional ratings with AI
Traditional measures of emotional responses have relied on methods such as brain responses (e.g., EEG and fMRI), pupil dilation (pupils respond both to light, cognitive load, and emotional events), pulse and respiration, and skin conductance. Most of these measures track arousal only, while a few can assess both dimensions. These are the foundation for all neuromarketing measures of emotions today. But new methods are on the horizon.
Our work focused on the last few seconds of each music video
In a paper recently presented at the 19th IEEE International Conference on Bioinformatics and Bioengineering (BIBE), we presented the results of new work where we combined EEG with machine learning to identify the two main dimensions of emotions (see the PDF here). Basically, we used EEG data to test whether it was possible to track emotional arousal and valence to music videos. In particular, we wanted to test whether we could use EEG responses during the last-second changes of music videos to predict later ratings of emotional valence and intensity. Our research question pertains to the so-called peak-end rule, in which our emotions at the peak and the end of an event tend to dominate the way in which we rate the whole event, although we focused on the end EEG responses only.
So, could EEG responses during the last seconds of music videos predict subsequent ratings? In the study, we trained an unsupervised machine learning model to identify music videos both in terms of their emotional arousal and valence and in clustering the music videos in terms of high and low performing videos. Here, we found that both goals could be met. First, we could successfully rate videos in terms of their emotional valence and arousal from EEG responses during the last few seconds in each video. Second, we successfully classified music videos in terms of whether they had low or high performance among our participants.
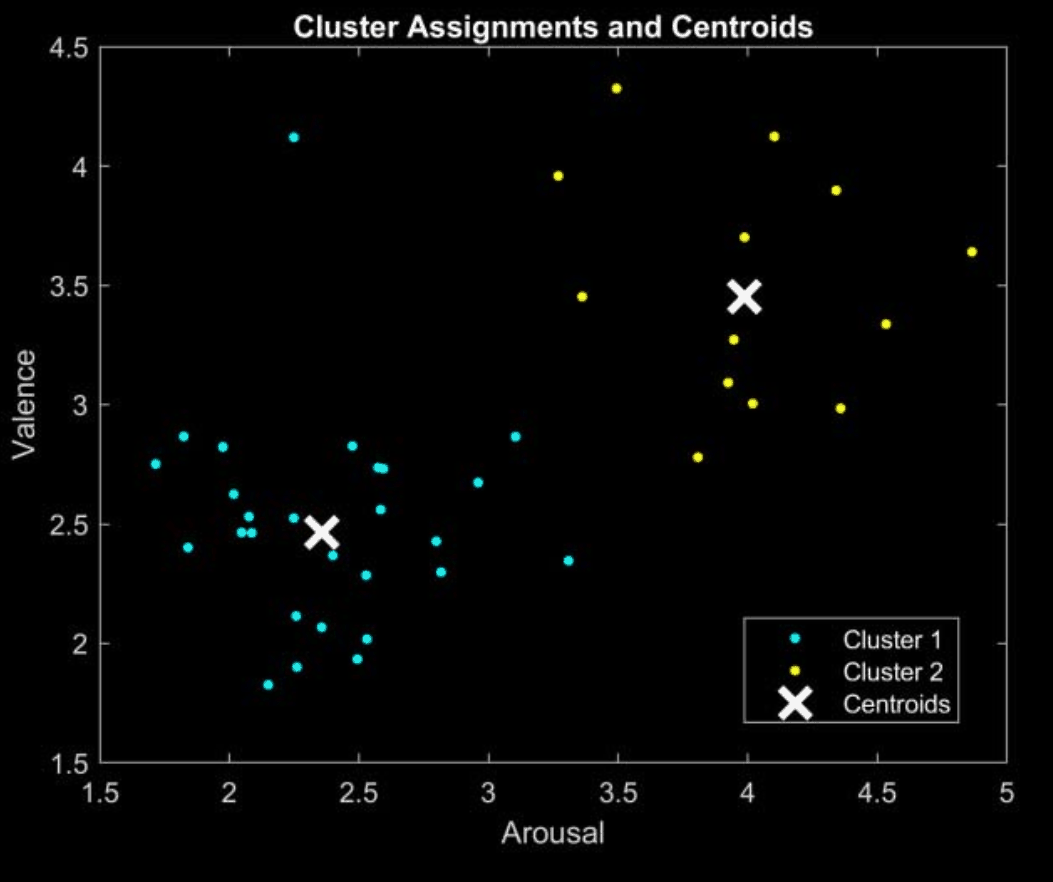
Interestingly, an additional premise we set out to achieve was to make these predictions using a user-independent method. This step is crucial, as we often see large individual differences in any brain responses, which may be due to gender, age, or other reasons. Here, our model success suggested that we could indeed make predictions in a user-independent manner, thus allowing our approach to become generally applicable outside of this single study.
Next steps for AI and neuromarketing?
The present paper constitutes the first steps in creating a predictive score of consumer preference and action. While the present work focused on music video preference, the same approach can in principle be applied to other consumer behaviors, such as movies and trailers, restaurant visits, and other consumption behaviors. Neurons also constantly works on improving our methods and metrics. This work represents our next level of approach, where we specifically use the database of thousands of people we have tested over the past years, and in driving better existing metrics, as well as completely new metrics.
As with any machine learning approach, the algorithmic model is only part of the solution: having a large and labeled data set is among the most important assets one can have. The Neurons NeuroMetric database is among the largest of its kind in this industry, allowing us to probe deeper and broader into new solutions. There is definitely more to come, so stay tuned!